3. Feature postprocessing from paper.¶
Title: “Predicting yeast synthetic lethal genetic interactions using protein domains”
Authors: Bo Li, Feng Luo,School of Computing,Clemson University,Clemson, SC, USA
e-mail: bol, luofeng@clemson.edu
year:2009
import pandas as pd
import numpy as np
import matplotlib.pyplot as plt
from collections import defaultdict
import seaborn as sns
import matplotlib.cm as cm
import scipy as scipy
import random
3.1. Importing datasets¶
3.1.1. Link to the github repo where the datasets to be downloaded:¶
import os
script_dir = os.path.dirname('__file__') #<-- absolute dir the script is in
rel_path_SL = "datasets/data-synthetic-lethals.xlsx"
rel_path_nSL="datasets/data-positive-genetic.xlsx"
rel_path_domains="datasets/proteins-domains-from-Pfam.xlsx"
abs_file_path_SL = os.path.join(script_dir, rel_path_SL)
abs_file_path_nSL = os.path.join(script_dir, rel_path_nSL)
abs_file_path_domains = os.path.join(script_dir, rel_path_domains)
# os.chdir('mini_book/docs/') #<-- for binder os.chdir('../')
# os.chdir('../')
my_path_sl= abs_file_path_SL
my_path_non_sl=abs_file_path_nSL
my_path_domains=abs_file_path_domains
data_sl=pd.read_excel(my_path_sl,header=0)
data_domains=pd.read_excel(my_path_domains,header=0,index_col='Unnamed: 0')
data_domains=data_domains.dropna()
data_nonsl=pd.read_excel(my_path_non_sl,header=0)
3.2. Building the feature matrix¶
One matrix for true SL where each row is one pair of SL. Every raw will be a vector of 0,1 or 2 depending on the comparison with the domain list. For row i the jth element = 0 if the jth element of the domain list is not in neither protein A and B, 1, if it is in one of them and 2 if it is in both of them .
3.2.1. Building the list of proteins domains id per protein pair separately :¶
List of protein A: Search for the Sl/nSL database the query gene name and look in the protein domain database which protein domains id has each of those queries.
List of protein B: Search for the Sl/nSL database the target gene name of the previous query and look in the protein domain database which protein domains id has each of those target genes.
# Selecting the meaningful columns in the respective dataset
domain_id_list=data_domains['domain-name']
query_gene=data_sl['gene-query-name']
target_gene=data_sl['gene-target-name']
query_gene_nonlethal=data_nonsl['gene-query-name']
target_gene_nonlethal=data_nonsl['gene-target-name']
# Initialising the arrays
protein_a_list=[]
protein_b_list=[]
protein_a_list_non=[]
protein_b_list_non=[]
population = np.arange(0,len(data_sl))
# For loop for 10000 pairs sampled randomly from the SL/nSl pair list , and creating a big array of proteind domains id per protein pair
for m in random.sample(list(population), 500):
protein_a=data_domains[data_domains['name']==query_gene[m]]
protein_b=data_domains[data_domains['name']==target_gene[m]]
protein_a_list.append(protein_a['domain-name'].tolist())
protein_b_list.append(protein_b['domain-name'].tolist())
protein_a_non=data_domains[data_domains['name']==query_gene_nonlethal[m]]
protein_b_non=data_domains[data_domains['name']==target_gene_nonlethal[m]]
protein_a_list_non.append(protein_a_non['domain-name'].tolist())
protein_b_list_non.append(protein_b_non['domain-name'].tolist())
print('We are going to analyze',len((protein_a_list)) ,'protein pairs, out of',len(data_sl),'SL protein pairs')
print('We are going to analyze',len((protein_a_list_non)) ,'protein pairs, out of',len(data_nonsl),'positive protein pairs')
We are going to analyze 500 protein pairs, out of 17871 SL protein pairs
We are going to analyze 500 protein pairs, out of 43340 positive protein pairs
3.2.2. Postprocessing #1: Remove protein pairs from study if either protein in the pair does not contain any domain¶
def remove_empty_domains(protein_list_search,protein_list_pair):
index=[]
for i in np.arange(0,len(protein_list_search)):
if protein_list_search[i]==[] or protein_list_pair[i]==[]:
index.append(i) ## index of empty values for the protein_a_list meaning they dont have any annotated domain
y=[x for x in np.arange(0,len(protein_list_search)) if x not in index] # a list with non empty values from protein_a list
protein_list_search_new=[]
protein_list_pair_new=[]
for i in y:
protein_list_search_new.append(protein_list_search[i])
protein_list_pair_new.append(protein_list_pair[i])
return protein_list_search_new,protein_list_pair_new
## evaluating the function
protein_a_list_new,protein_b_list_new=remove_empty_domains(protein_a_list,protein_b_list)
protein_a_list_non_new,protein_b_list_non_new=remove_empty_domains(protein_a_list_non,protein_b_list_non)
print('The empty domain in the SL were:', len(protein_a_list)-len(protein_a_list_new), 'out of', len(protein_a_list),'domains')
print('The empty domain in the nSL were:', len(protein_a_list_non)-len(protein_a_list_non_new), 'out of', len(protein_a_list_non),'domains')
The empty domain in the SL were: 86 out of 500 domains
The empty domain in the nSL were: 100 out of 500 domains
3.2.3. Feature engineering: Select from each ordered indexes of domain id list which of them appear once, in both or in any of the domains of each protein pair¶
3.2.3.1. Define function get_indexes
¶
get_indexes = lambda x, xs: [i for (y, i) in zip(xs, range(len(xs))) if x == y] # a function that give the index of whether a value appear in array or not
a=[1,2,2,4,5,6,7,8,9,10]
get_indexes(2,a)
[1, 2]
def feature_building(protein_a_list_new,protein_b_list_new):
x = np.unique(domain_id_list)
## To avoid taking repeated domains from one protein of the pairs , lets reduced the domains of each protein from the pairs to their unique members
protein_a_list_unique=[]
protein_b_list_unique=[]
for i in np.arange(0,len(protein_a_list_new)):
protein_a_list_unique.append(np.unique(protein_a_list_new[i]))
protein_b_list_unique.append(np.unique(protein_b_list_new[i]))
protein_feat_true=np.zeros(shape=(len(x),len(protein_a_list_unique)))
pair_a_b_array=[]
for i in np.arange(0,len(protein_a_list_unique)):
index_a=[]
pair=[protein_a_list_unique[i],protein_b_list_unique[i]]
pair_a_b=np.concatenate(pair).ravel()
pair_a_b_array.append(pair_a_b)
j=0
for i in pair_a_b_array:
array,index,counts=np.unique(i,return_index=True,return_counts=True)
for k,m in zip(counts,array):
if k ==2:
protein_feat_true[get_indexes(m,x),j]=2
if k==1:
protein_feat_true[get_indexes(m,x),j]=1
j=j+1
return protein_feat_true
protein_feat_true=feature_building(protein_b_list_new=protein_b_list_new,protein_a_list_new=protein_a_list_new)
protein_feat_true_pd=pd.DataFrame(protein_feat_true.T)
protein_feat_non_true=feature_building(protein_b_list_new=protein_b_list_non_new,protein_a_list_new=protein_a_list_non_new)
protein_feat_non_true_pd=pd.DataFrame(protein_feat_non_true.T)
3.2.4. How many ones and twos are in each dataset¶
index_2_true=protein_feat_true_pd.where(protein_feat_true_pd==2)
index_2_true_count=index_2_true.count(axis=1).sum()
index_1_true=protein_feat_true_pd.where(protein_feat_true_pd==1)
index_1_true_count=index_1_true.count(axis=1).sum()
index_2_nontrue=protein_feat_non_true_pd.where(protein_feat_non_true_pd==2)
index_2_nontrue_count=index_2_nontrue.count(axis=1).sum()
index_1_nontrue=protein_feat_non_true_pd.where(protein_feat_non_true_pd==1)
index_1_nontrue_count=index_1_nontrue.count(axis=1).sum()
print('fraction of twos in the SL array is',index_2_true_count/(len(protein_feat_true_pd.index)*len(protein_feat_true_pd.columns)))
print('fraction of ones in the SL array is',index_1_true_count/(len(protein_feat_true_pd.index)*len(protein_feat_true_pd.columns)))
print('fraction of twos in the PI array is',index_2_nontrue_count/(len(protein_feat_non_true_pd.index)*len(protein_feat_non_true_pd.columns)))
print('fraction of ones in the PI array is',index_1_nontrue_count/(len(protein_feat_non_true_pd.index)*len(protein_feat_non_true_pd.columns)))
fraction of twos in the SL array is 2.7148959955284064e-05
fraction of ones in the SL array is 0.0009757655607457979
fraction of twos in the PI array is 6.611570247933884e-06
fraction of ones in the PI array is 0.0008702479338842975
3.2.4.1. Bar plot to visualize these numbers¶
plt.bar(['fraction of 2 in the nSL','fraction of 1 in the nSL'],[index_2_nontrue_count/(len(protein_feat_non_true_pd.index)*len(protein_feat_non_true_pd.columns)),index_1_nontrue_count/(len(protein_feat_non_true_pd.index)*len(protein_feat_non_true_pd.columns))],alpha=0.6,color=['blue','lightblue']),
plt.bar(['fraction of 2 in SL ','fraction of 1 in SL'],[index_2_true_count/(len(protein_feat_true_pd.index)*len(protein_feat_true_pd.columns)),index_1_true_count/(len(protein_feat_true_pd.index)*len(protein_feat_true_pd.columns))],alpha=0.6,color=['coral','lightcoral'])
plt.ylabel('Fraction from the population')
plt.yscale('log')
plt.xticks(rotation=40)
([0, 1, 2, 3], <a list of 4 Text xticklabel objects>)
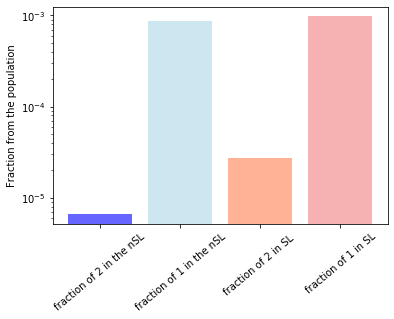
3.2.4.2. Adding the labels(response variables) to each dataset¶
protein_feat_true_pd['lethality']=np.ones(shape=(len(protein_a_list_new)))
protein_feat_non_true_pd['lethality']=np.zeros(shape=(len(protein_a_list_non_new)))
3.2.4.3. Joining both datasets¶
feature_post=pd.concat([protein_feat_true_pd,protein_feat_non_true_pd],axis=0)
feature_post=feature_post.set_index(np.arange(0,len(protein_a_list_new)+len(protein_a_list_non_new)))
print('The number of features are:',feature_post.shape[1])
print('The number of samples are:',feature_post.shape[0])
The number of features are: 3026
The number of samples are: 814
3.2.5. Postprocessing and exploration of the feature matrix of both datasets¶
mean=feature_post.T.describe().loc['mean']
std=feature_post.T.describe().loc['std']
lethality=feature_post['lethality']
corr_keys=pd.concat([mean,std,lethality],axis=1)
3.2.6. Viz of the stats¶
pair=sns.pairplot(corr_keys,hue='lethality',diag_kind='kde',kind='reg',palette='colorblind')
pair.fig.suptitle('Pairplot to see data dependencies with Lethality',y=1.08)
Text(0.5, 1.08, 'Pairplot to see data dependencies with Lethality')
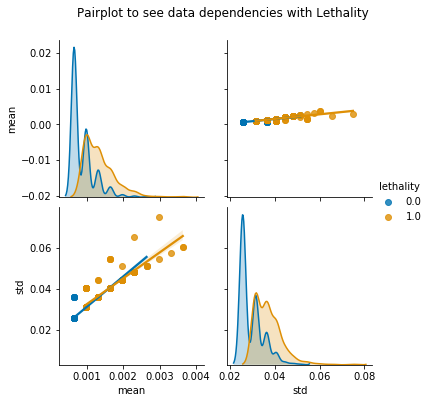
X, y = feature_post.drop(columns=["lethality"]), feature_post["lethality"]
from sklearn.preprocessing import StandardScaler
scaler = StandardScaler()
x_sl=protein_feat_true_pd.drop(columns=['lethality'])
x_nsl=protein_feat_non_true_pd.drop(columns=['lethality'])
X_sl_scaled = scaler.fit_transform(x_sl)
X_nsl_scaled = scaler.fit_transform(x_nsl)
np.shape(X_sl_scaled)
(414, 3025)
3.3. How redundant are each of the protein domains?¶
def PCA_component_contribution(scaled_matrix,original_data):
from sklearn.decomposition import PCA
model = PCA(0.95).fit(scaled_matrix)
## apply dimensionality reduction to X_train
output_pca = model.transform(scaled_matrix)
total=sum(model.explained_variance_)
# number of components , that it will be the number of main axes times the number of original components
n_pcs= model.components_.shape[0] # the amount of non redundant protein domains
# get the index of the most important feature on EACH component
# LIST COMPREHENSION HERE
most_important = [np.abs(model.components_[i]).argmax() for i in range(n_pcs)]
initial_feature_names = original_data.columns
# get the names
most_important_names = [initial_feature_names[most_important[i]] for i in range(n_pcs)]
# LIST COMPREHENSION HERE AGAIN
dic = {'PC{}'.format(i): most_important_names[i] for i in range(n_pcs)}
# build the dataframe
df = pd.DataFrame(dic.items(),columns=['pca-component','domain-number'])
return df,model.components_
df_sl,components_pca_nsl=PCA_component_contribution(X_nsl_scaled,x_nsl)
df_nsl,components_pca_sl=PCA_component_contribution(X_sl_scaled,x_sl)
df_sl.head()
pca-component | domain-number | |
---|---|---|
0 | PC0 | 467 |
1 | PC1 | 2 |
2 | PC2 | 339 |
3 | PC3 | 754 |
4 | PC4 | 219 |
3.5. Domains exclusive to SL¶
df_sl_exclusive=pd.merge(df_sl,df_nsl,how='left',on='domain-number')
domains_name=np.unique(data_domains['domain-name'])
domains_description=np.unique(data_domains['domain-descrip'])
df_sl_exclusive['domain-description']=domains_description[df_sl_exclusive['domain-number']]
df_sl_exclusive[pd.isnull(df_sl_exclusive['pca-component_y'])]
pca-component_x | domain-number | pca-component_y | domain-description | |
---|---|---|---|---|
0 | PC0 | 467 | NaN | Choline_transpo; Choline transporter-like |
2 | PC2 | 339 | NaN | CDC50; CDC50/LEM3 family |
3 | PC3 | 754 | NaN | Diphthamide_syn; Diphthamide synthesis DPH1/DPH2 |
4 | PC4 | 219 | NaN | Apc15p; Anaphase-promoting complex subunit 15... |
5 | PC5 | 134 | NaN | ATP-synt_ab_N; ATPase, F1/V1/A1 complex, alpha... |
... | ... | ... | ... | ... |
271 | PC260 | 778 | NaN | E1_UFD; Ubiquitin-activating enzyme E1, C-term... |
273 | PC262 | 2952 | NaN | tRNA_int_end_N2; tRNA-splicing endonuclease, s... |
274 | PC263 | 1148 | NaN | HUN; Hpc2-related domain |
275 | PC264 | 299 | NaN | Bmt2; 25S rRNA (adenine(2142)-N(1))-methyltran... |
276 | PC265 | 1089 | NaN | Guanylate_kin; Guanylate kinase/L-type calcium... |
216 rows × 4 columns
print('The number of exclusive domains from SL pairs, after PCA, is = ',len(df_sl_exclusive), 'out of', len(df_sl)+len(df_nsl), 'so the',100*len(df_sl_exclusive)/(len(df_sl)+len(df_nsl)),'%')
The number of exclusive domains from SL pairs, after PCA, is = 277 out of 536 so the 51.67910447761194 %
df_nsl_exclusive=pd.merge(df_sl,df_nsl,how='right',on='domain-number')
domains_name=np.unique(data_domains['domain-name'])
domains_description=np.unique(data_domains['domain-descrip'])
df_nsl_exclusive['domain-description']=domains_description[df_nsl_exclusive['domain-number']]
df_nsl_exclusive[pd.isnull(df_nsl_exclusive['pca-component_x'])]
pca-component_x | domain-number | pca-component_y | domain-description | |
---|---|---|---|---|
61 | NaN | 1028 | PC0 | GatB_Yqey; Asn/Gln amidotransferase |
62 | NaN | 531 | PC1 | Cyclin_C; Cyclin, C-terminal domain |
63 | NaN | 101 | PC2 | ARD; Acireductone dioxygenase ARD family |
64 | NaN | 268 | PC3 | BHD_3; Rad4 beta-hairpin domain 3 |
65 | NaN | 2113 | PC5 | Rav1p_C; RAVE complex protein Rav1 C-terminal |
... | ... | ... | ... | ... |
278 | NaN | 482 | PC262 | Clathrin_propel; Clathrin, heavy chain, propel... |
279 | NaN | 2568 | PC264 | TEA; TEA/ATTS domain |
280 | NaN | 2524 | PC266 | Sterol-sensing; Sterol-sensing domain |
281 | NaN | 1509 | PC268 | Motile_Sperm; Major sperm protein (MSP) domain |
282 | NaN | 2137 | PC269 | Rib_5-P_isom_A; Ribose 5-phosphate isomerase, ... |
222 rows × 4 columns
print('The number of exclusive domains from non SL pairs, after PCA, is = ',len(df_nsl_exclusive), 'out of', len(df_sl)+len(df_nsl), 'so the',100*len(df_nsl_exclusive)/(len(df_sl)+len(df_nsl)),'%')
The number of exclusive domains from non SL pairs, after PCA, is = 283 out of 536 so the 52.798507462686565 %
3.6. Let see to the domains-number that get repeated after the PCA analysis , and selecting the most explanatory feature by its explained variance.¶
duplicated_features_sl=df_sl[df_sl.iloc[:,1].duplicated()]
repeated_features_sl=pd.DataFrame()
domains_name=np.unique(data_domains['domain-name'])
domains_description=np.unique(data_domains['domain-descrip'])
repeated_features_sl['domain-name']=domains_name[duplicated_features_sl.iloc[:,1]]
repeated_features_sl['domain-description']=domains_description[duplicated_features_sl.iloc[:,1]]
duplicated_features_nsl=df_nsl[df_nsl.iloc[:,1].duplicated()]
repeated_features_nsl=pd.DataFrame()
repeated_features_nsl['domain-name']=domains_name[duplicated_features_nsl.iloc[:,1]]
repeated_features_nsl['domain-description']=domains_description[duplicated_features_nsl.iloc[:,1]]
only_sl_pd=pd.merge(repeated_features_sl,repeated_features_nsl,how='left',on=['domain-name'])
data_only_sl = only_sl_pd[pd.isnull(only_sl_pd['domain-description_y'])]
data_only_sl['domain-description']=data_only_sl['domain-description_x']
data_only_sl.shape,df_sl_exclusive.shape
c:\users\linigodelacruz\appdata\local\continuum\anaconda3\envs\wintest\lib\site-packages\ipykernel_launcher.py:3: SettingWithCopyWarning:
A value is trying to be set on a copy of a slice from a DataFrame.
Try using .loc[row_indexer,col_indexer] = value instead
See the caveats in the documentation: http://pandas.pydata.org/pandas-docs/stable/user_guide/indexing.html#returning-a-view-versus-a-copy
This is separate from the ipykernel package so we can avoid doing imports until
((40, 4), (277, 4))
only_nsl_pd=pd.merge(repeated_features_sl,repeated_features_nsl,how='right',on=['domain-name'])
data_only_nsl = only_nsl_pd[pd.isnull(only_nsl_pd['domain-description_x'])]
data_only_nsl
domain-name | domain-description_x | domain-description_y | |
---|---|---|---|
3 | PF02714 | NaN | Fmp27_SW; FMP27, SW domain |
4 | PF13634 | NaN | Thioredoxin_7 |
5 | PF09088 | NaN | RSF; Respiration factor 1 |
6 | PF00580 | NaN | BRCT_2; BRCT domain |
7 | PF16589 | NaN | eIF-6; Translation initiation factor IF6 |
8 | PF13401 | NaN | TPR_6; Tetratricopeptide repeat |
9 | PF04100 | NaN | Kre28; Spindle pole body component Kre28 |
10 | PF00175 | NaN | ANAPC10; APC10/DOC domain |
11 | PF00181 | NaN | ANAPC4_WD40; Anaphase-promoting complex subuni... |
12 | PF00736 | NaN | CLTH; CTLH/CRA C-terminal to LisH motif domain |
13 | PF04049 | NaN | Isy1; Pre-mRNA-splicing factor Isy1 |
14 | PF00176 | NaN | ANAPC1; Anaphase-promoting complex subunit 1 |
15 | PF00298 | NaN | Abhydrolase_2; Phospholipase/carboxylesterase/... |
16 | PF10681 | NaN | SLC12; SLC12A transporter, C-terminal |
17 | PF10452 | NaN | SDA1; SDA1 domain |
18 | PF01302 | NaN | DJ-1_PfpI; DJ-1/PfpI |
19 | PF11488 | NaN | SPT2; Chromatin SPT2 |
20 | PF08690 | NaN | RNA_pol_Rpb2_7; RNA polymerase Rpb2, domain 7 |
21 | PF12090 | NaN | Sec61_beta; Protein transport protein SecG/Sec... |
22 | PF00266 | NaN | ATP-synt_J; ATP synthase, F0 complex, subunit J |
23 | PF00533 | NaN | BAR_2; BAR domain-containing family |
24 | PF12726 | NaN | Steroid_dh; 3-oxo-5-alpha-steroid 4-dehydrogen... |
25 | PF01115 | NaN | CoaE; Dephospho-CoA kinase |
26 | PF03371 | NaN | HBS1_N; HBS1-like protein, N-terminal |
27 | PF08144 | NaN | PRO8NT; PRO8NT domain |
28 | PF08243 | NaN | Pept_tRNA_hydro; Peptidyl-tRNA hydrolase |
29 | PF02383 | NaN | FHA; Forkhead-associated (FHA) domain |
30 | PF02996 | NaN | Gal_mutarotas_2; Glycoside hydrolase family 31... |
31 | PF00957 | NaN | Candida_ALS_N; Agglutinin-like protein, N-term... |
32 | PF07393 | NaN | PCRF; Peptide chain release factor |
33 | PF07393 | NaN | PCRF; Peptide chain release factor |
34 | PF08118 | NaN | PRKCSH_1; Mannose-6-phosphate receptor binding... |
35 | PF00399 | NaN | Alpha_adaptinC2; Clathrin adaptor, alpha/beta/... |
36 | PF00233 | NaN | ATG22; Autophagy-related protein 22-like |
37 | PF10156 | NaN | Ribosomal_L37; Ribosomal protein L37, mitochon... |
38 | PF04281 | NaN | Lsm_interact; LSM-interacting domain |
39 | PF10642 | NaN | SKIP_SNW; SKI-interacting protein SKIP, SNW do... |
40 | PF14475 | NaN | UDPGT; UDP-glucuronosyl/UDP-glucosyltransferase |
41 | PF00620 | NaN | Brix; Brix domain |
42 | PF00995 | NaN | Cation_efflux; Cation efflux protein |
43 | PF01151 | NaN | Cpn10; GroES chaperonin family |
shared_domains_pd=pd.merge(repeated_features_sl,repeated_features_nsl,how='inner',on=['domain-name'])
shared_domains_pd
domain-name | domain-description_x | domain-description_y | |
---|---|---|---|
0 | PF01909 | Dynein_light; Dynein light chain, type 1/2 | Dynein_light; Dynein light chain, type 1/2 |
1 | PF00439 | Anth_synt_I_N; Anthranilate synthase component... | Anth_synt_I_N; Anthranilate synthase component... |
2 | PF00439 | Anth_synt_I_N; Anthranilate synthase component... | Anth_synt_I_N; Anthranilate synthase component... |
a_sl=x_sl.iloc[:,np.sort(df_sl.iloc[:,1])]
a_sl.describe().loc['mean'].hist(bins=8),
a_sl.describe().loc['std'].hist(bins=8,alpha=0.4)
plt.xlim([0,0.2])
(0, 0.2)
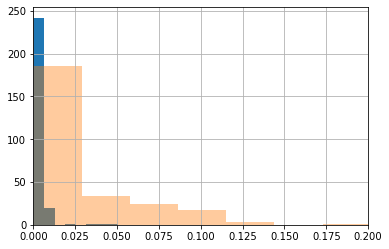
a_nsl=x_nsl.iloc[:,np.sort(df_nsl.iloc[:,1])]
a_nsl.describe().loc['mean'].hist(bins=8),
a_nsl.describe().loc['std'].hist(bins=8,alpha=0.4)
plt.xlim([0,0.2])
(0, 0.2)
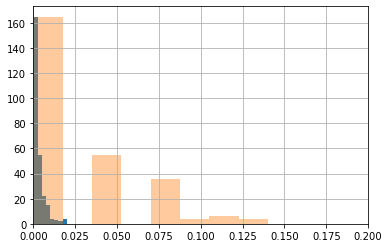
3.6.1. Proof of concept with BEM1¶
Gather the domains of SL and nSL pairs from BEM1
Check if the domains for the SL and nSL pairs are inside the most important domains after PCA.
domains_bem1=data_domains[data_domains['name']=='BEM1']['domain-descrip']
sl_bem1=data_sl[data_sl['gene-query-name']=='BEM1']['gene-target-name']
sl_bem1
2846 BEM2
2847 CDC24
5004 MSB1
13777 SMI1
13778 BNI1
13779 BEM2
14385 SKN7
Name: gene-target-name, dtype: object
data_sl[data_sl['gene-query-name']=='BEM1']
gene-query | gene-target | gene-query-name | gene-target-name | gene-query-description | gene-target-description | interaction-type | paper-source | |
---|---|---|---|---|---|---|---|---|
2846 | YBR200W | YER155C | BEM1 | BEM2 | SRO1|phosphatidylinositol-3-phosphate-binding ... | IPL2|SUP9|TSL1|L000000168 | Synthetic Lethality | Peterson J (1994) |
2847 | YBR200W | YAL041W | BEM1 | CDC24 | SRO1|phosphatidylinositol-3-phosphate-binding ... | CLS4|Rho family guanine nucleotide exchange fa... | Synthetic Lethality | Peterson J (1994) |
5004 | YBR200W | YOR188W | BEM1 | MSB1 | SRO1|phosphatidylinositol-3-phosphate-binding ... | L000001184 | Synthetic Lethality | Bender A (1991) |
13777 | YBR200W | YGR229C | BEM1 | SMI1 | SRO1|phosphatidylinositol-3-phosphate-binding ... | KNR4|L000000909 | Synthetic Lethality | Gorelik M (2011) |
13778 | YBR200W | YNL271C | BEM1 | BNI1 | SRO1|phosphatidylinositol-3-phosphate-binding ... | PPF3|SHE5|formin BNI1|L000000190 | Synthetic Lethality | Gorelik M (2011) |
13779 | YBR200W | YER155C | BEM1 | BEM2 | SRO1|phosphatidylinositol-3-phosphate-binding ... | IPL2|SUP9|TSL1|L000000168 | Synthetic Lethality | Gorelik M (2011) |
14385 | YBR200W | YHR206W | BEM1 | SKN7 | SRO1|phosphatidylinositol-3-phosphate-binding ... | BRY1|POS9|kinase-regulated stress-responsive t... | Dosage Lethality | Bouquin N (1999) |